The Transformative Impact of AI on Healthcare
- younginnovatorsste
- Jun 27, 2024
- 5 min read
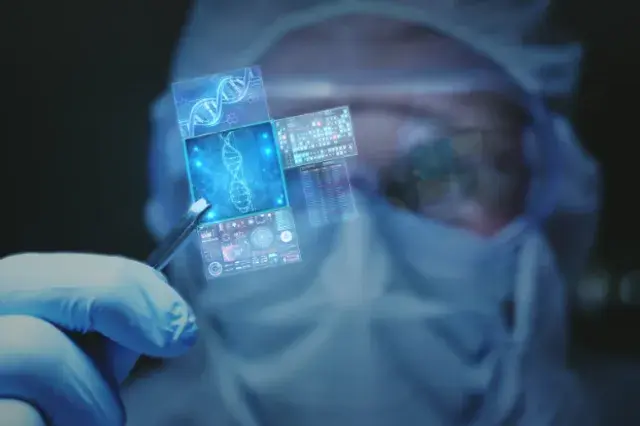
By: Max Yuan
In the ever-evolving landscape of technology, few innovations have shown as much promise and potential as artificial intelligence (AI). From transforming industries to reshaping daily lives, AI's influence has been remarkable, and nowhere is this more evident than in the field of medicine. The convergence of AI and healthcare has unleashed a wave of advancements that are revolutionizing patient care, diagnosis, treatment, and research [1].
Let’s Talk About AI and Healthcare’s History First
AI has made significant strides in patient diagnosis throughout, particularly in visually-oriented specialties like dermatology. Researchers have developed classification models, such as deep convolutional neural networks (DCNN), to aid dermatologists in diagnosing skin conditions like cancer and psoriasis [1]. For instance, Andre Esteva and Achim Hekler trained a DCNN using over 129,000 images to classify skin cancers with performance comparable to 21 board-certified dermatologists. Similarly, AI has advanced patient prognosis, as seen with Google's DCNN, and can classify diabetic retinopathy and macular edema using retinal fundus images [1]. This AI model not only enhances efficiency and offers a second opinion but also detects early-stage diabetic retinopathy and expands screening coverage. Beyond diagnosis and prognosis, AI has found application in drug discovery and personalized treatment options, addressing diseases like Parkinson's and Alzheimer's. AI systems like BotMD were developed to enhance patient care, offering 24/7 clinical support and assisting with appointments, prescription inquiries, and hospital protocol searches [1]. As AI continues to transform healthcare, its potential to revolutionize patient care and medical processes is evident.
What Types of AI Tech are Important in Healthcare?
Artificial intelligence (AI) encompasses a spectrum of technologies with profound implications for healthcare, extending beyond singular definitions. While their immediate relevance to medicine is evident, the array of processes they empower is extensive. At the forefront is machine learning, characterized by neural networks and deep learning, enabling adaptation through iterative data exposure. Its prevalence is shown by the 63% adoption rate of machine learning among US organizations pursuing AI in 2018 [2].
In healthcare, machine learning finds significant application in precision medicine, predicting optimal treatment protocols based on patient attributes. Neural networks, dating back to the 1960s, and deep learning, with multi-layered models predicting outcomes, are pivotal components. They excel in tasks like discerning malignant lesions in radiology images and detecting clinically significant features. Natural language processing (NLP), developed since the 1950s, powers speech recognition, text analysis, and conversational AI in healthcare, crafting, comprehending, and classifying clinical documentation [1]. The integration of AI-driven robots spans tangible and process-driven realms, from industrial robots executing tasks to surgical robots enhancing precision in minimally invasive procedures. Robotic process automation (RPA) streamlines administrative functions enriched by image recognition—AI's trajectory in diagnosis and treatment dates to the 1970s, with the likes of MYCIN. However, integration and performance issues hindered early rule-based systems, while IBM's Watson garnered attention for precision medicine despite integration challenges [1]. Tech firms and startups drive AI applications, collaborating on predictive models and diagnosis enhancements. Despite these advancements, integrating AI-driven diagnosis and treatment into clinical workflows remains complex [4]. In a healthcare landscape transformed by AI, the fusion of machine learning, robotics, and NLP blurs boundaries, manifesting in precision medicine, surgical enhancement, and administrative efficiency. A paradigm shift towards AI-fortified clinical decision-making is underway.
Problems Concerning AI
As AI systems integrate into diverse domains, including healthcare, several challenges arise. As people explore these challenges and focus on those pertinent to healthcare, shifting from a reactive to a proactive approach becomes hugely crucial to navigating this technological evolution. In particular, constructing effective AI systems hinges on robust data collection, and strong models demand substantial-high-quality data. However, the more significant amounts of data collection required by AI software sparks problems driven by patient privacy concerns and corporate data breaches. Technological advances amplify computational power and, therefore, data storage, enabling technologies like facial recognition and gene analysis for individual identification that require megabytes of storage on standard computers. These innovations that uphold patient privacy and data control are paramount to prevent breaches that could lead to denied medical coverage.
Limited data access hurts model training, restricting potential—bias in training data yields biased models, underscoring the need for representative data collection [3]. Even unbiased data requires careful preprocessing to avoid introducing bias. Model selection is pivotal, requiring precise alignment with task demands [3]. Users must comprehend model-building processes for accurate interpretation of outputs. Data sharing constraints among healthcare organizations disrupt seamless model transfer. Privacy concerns lead to fragmented data landscapes, weakening model reliability. The complexity of AI algorithms often obscures transparency, prompting efforts to enhance accessibility and interpretability [3].
There has also been a problem concerning healthcare workers and their jobs as they fear that AI will replace them [4]. However, this has been dealt with as companies and employers have said that they need workers trained with the knowledge that physicians and data scientists need to have to develop such technology that deals with the lives of others. Physicians must comprehend AI's potential and assess its impact on their roles, conveying this to data scientists for system development.
Future of AI’s Impact on Healthcare
While AI in healthcare has limitations, its potential to revolutionize the industry is significant. AI systems can enhance doctors' efficiency by transcribing notes, organizing patient data, diagnosing patients, and offering second opinions. Moreover, AI aids patients in follow-up care, prescription alternatives, and remote diagnoses, extending medical services to underserved areas [5]. Recent FDA approvals for devices like smartwatches highlight the AI potential for personal health data collection [2]. Virtual human interactions show promise to aid mental health discussions, enabling more accurate diagnosis and treatment. While AI has been invested in disease drug development, neglected tropical diseases and rare diseases have been overlooked [2]. Incorporating AI into medical education is crucial, given the complex decisions it involves. However, the need for standardized and well-represented data is a barrier to realizing AI's full potential in healthcare. The primary hurdle lies in integrating AI into daily clinical practice, requiring regulatory approval, EHR system integration, standardization, clinician training, funding, and ongoing updates. AI is envisioned as an augmentation to human clinicians, enhancing patient care by allowing healthcare professionals to focus on uniquely human skills [5]. In contrast, those who resist working alongside AI may be the only ones at risk of job displacement.
-----------------------------------------------------------------------------------------------------------------------
Author Max Yuan is an 12th grader from the Eastern United States, specializing in researching bioengineering and medical science topics.
Works Cited:
“Artificial Intelligence: How is It Changing Medical Sciences and Its Future?” PMC-NCBI. https://www.ncbi.nlm.nih.gov/pmc/articles/PMC7640807/
“The Potential for Artificial Intelligence in Healthcare” PMC-NCBI. https://www.ncbi.nlm.nih.gov/pmc/articles/PMC6616181/
“How AI And Machine Learning Will Impact The Future Of Healthcare” Forbes Magazine. https://www.forbes.com/sites/bernardmarr/2022/09/14/how-ai-and-machine-learning-will-impact-the-future-of-healthcare/
“Artificial Intelligence Beyond the Clinic” Harvard Medical School. https://hms.harvard.edu/news/artificial-intelligence-beyond-clinic
“Why is AI adoption in health care lagging?” Brookings. https://www.brookings.edu/articles/why-is-ai-adoption-in-health-care-lagging/
Comments